We are at the precipice of something totally, totally different with David Ramsey of ONIS Solutions
As CTO of ONIS Solutions, David Ramsey is at the forefront of the big data movement. With a passion for technology and nuanced view on the subject given his work in Africa, in this episode David shares how he expects data to change our lives in the future.
Citing industry examples such as food and transportation, David’s optimistic vision of the future imagines how data collection creates jobs and enables high net creativity.
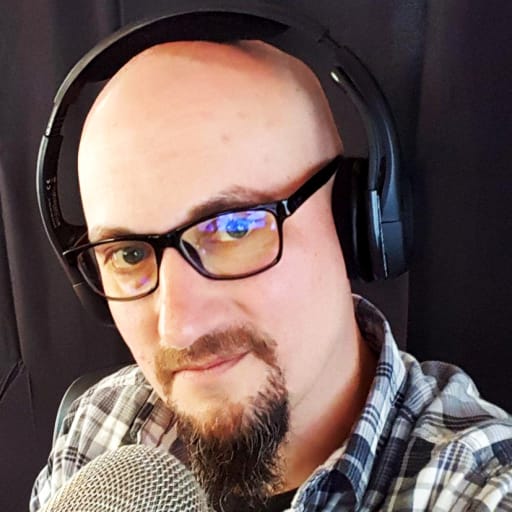
Transcript
Ledge: Hey, David. Really cool to have you on. Thanks for joining us.
David: Thanks, Ledge. It’s my pleasure to be here.
Ledge: Could you give two or three minute background story; you, your work, and what you’re into it, just so the listeners can get to know you a little bit?
David: Absolutely. Currently CTO of ONIS Solutions, that’s O-N-I-S not O-N-U-S. We’re a data and a telco-focused SI. We are driving towards AI implementation in the telco segment.
I came to this in a very indirect fashion. I was CEO of Jadora. It was a carbon offset company in the Democratic Republic of Congo, of all places. We were trying to create carbon offsets and sell them on the voluntary and involuntary market.
Of course, we ran into crazy political situations lately and we had the untimely passing of our CEO, Don Tuttle. It was untimely but it was a fantastic experience and one a lot of people don’t have. I spent a lot of time in country and a lot of time here. I spent some time at Microsoft. My last role was, I ran OEM sales out of Dubai. So I got to see the higher part of the disposable income in Africa and some of the very bottom poverty levels. It was a life changing experience, to be honest.
Had a tour duty also at HPE and AT&T Wireless. My formal background in education is PhD in Chemical Engineering at the University of Texas.
Ledge: Fantastic. Very cool experience at a worldwide there, where you learned so much about just different cultures and that tech ecosystem across the board.
David: Absolutely. It’s driving where my passion is today. I’ve always had a passion for technology, but when you see the segment of people that just simple things changed not just a life but a family, it really drives where you want to spend the next …, the next things you’re doing too.
Ledge: Prior to our discussion here before we were recording, you talked about the technology adoption of the next billion people. That’s a huge vision. We get Zuckerberg talking about things like that.
Just wondering, what’s your area of interest and thought process there from that futurist perspective?
David: Well, let me ask you a question. I’ll make three statements and tell me when this year is that we’re thinking of.
If I was to tell you that we have incoming quality has climbed, it’s at the highest levels ever. That medium wages and living standards have flattened and the middle class is stagnant. But we’re on the edge of a new technology and we all see it coming but we can’t see the actual impact yet. What year would you think we’re living in?
Ledge: It could be pre-industrial revolution, and I think you’d be somewhere in the 1940s, and I think you’re now.
David: You’re the first person ever to get that right. So the pre-industrial part is called the Engels’ pause, of course.
When people talk about all the issues we had today, that timeframe had no middle class. If you look at the time today, by 2020 the World Bank estimates half the population of this world will be classified as middle class. It varies country to country of course but that’s three-and-a-half to four billion people will be defined as middle class. That’s never before happened in the country.
You mentioned the first Industrial Revolution. We’ve had the second, which is the age of science and mass production. Third is really the rise of digital technology.
What I’m going towards the fourth revolution will be to focus on that middle class for the first time ever. Really be the fusion of digital and biological and physical… It’s not bounded by a country or region or border, and definitely no wall. There’s a three-and-a-half billion people that are in Maslow’s hierarchy, if you think about it that way, they’re at the age of enlightenment. They want to attain their personal goals and their personal best. That is the mass that one to one-and-a-half billion of folks 12, 13, 14 to 30, that cloud technologies and DNA and AI are pristine for.
Being in Africa at the bottom side at the top side, when I think about it that way, we are on the precipice of something that’s totally, totally different. For me, it’s incredibly exciting.
Ledge: So, what do we do with that, those of us in the technical industries? I imagine some people here that they see dollar signs, and other people will hear that and they say, “Social justice,” The divider of, okay, where do we go now?
David: It’ huge, obviously, and a lot of more smart people know much more about this than I do. I look at it strictly from a technology side. When I think about it, it’s the old principle, it’s just now data. That will be, where is data, what is data and what is its value?
Instead of being data powered like everybody thinks they’re data powered. It’s really, what is data driving? What is this data driving?
For us and for me, if you think about it, a great example would be autonomous vehicles. When they first started coming out in 2010 and 2009, ‘It’s going to change the transportation industry, change the transportation industry.” Well, yes but no. What it’s going to change is the insurance companies, mechanics, it’s going to change the Fed and SLED’s allocation of money for roads. It’s going to change safety. It’s going to change everything else around that.
So that one simple segment that everyone thought was going to be dramatically different will have impact well beyond that. That’s what will happen in this revolution.
I believe and what I’m passionate about is the AI side and the data side. I’m sure we’ll be going way beyond that, Ledge, but for me it’s around the data side.
As you go into this calendar year, you’re seeing a lot of terms pop up like data curation and data valuation, that really haven’t been used before. Everyone thought they knew the life cycle or they thought they knew the value of their data because Google told them that, this advertisement is worth this, or Amazon believes the subscriber is worth this. But that’s not really the case when you have these massive data lakes and massive databases and data warehouses. Over tens of tens of years with multiples and exponential data coming in.
For me, AI is only good data in, data out. So you can have the best data model or the best data models and neural networks that you want, but without the right data and knowing what the value of that information is, then how do you really know what the value of that output is?
Ledge: I read some study that I can’t remember where I saw it, but in an article that, you’ve got your… If you think of three types of data or archetypes of data. You’ve got your structured and you’ve got your sort of structured – so you’ve got your relational databases and you’ve got your, I don’t know, XML or JSON or something of that nature – proceeding in the unstructured direction. Then you have fully unstructured data.
Now, the growth pattern is that the fully unstructured data is on the exponential growth curve while the other two are just linear. Now we need to figure out, what do we do with this massive data collection of everything to make, not just sort of targeting ads out of it, but what do you take that and then make some sort of better contributions to the world?
David: Agreed. To use your example, if you think about a dataset, no matter how massive or small,, the dataset and the value to you as an individual and as a company has got really six key elements.
It has intrinsic value which is, how correct and complete. Kind of a boring block and tackling but it’s the fundamental elements of the data. There’s a cost and a risk part of it. What happens if I lose that data? There’s a market value; I could sell that data externally. There’s an economical value; how does it help my EBITA, my bottom line internally? There’s a performance data, how does it affect the business drivers of my company – really impact the business drivers? Then there’s the business value; how is it relevant to the mission and core business of a company?
It doesn’t really matter how big it is. If one doesn’t know that, then how in the world can you create a Watson model or a Python model or any other technical implementation and on top of that, to get a key result of your business? You can’t even define what the value of the data going into it is? Does that make sense?
Ledge: Yeah. I mean, you’ve got sort of an asset valuation model then for data. Which is an interesting paradigm because, you wouldn’t have thought prior that the data itself could be valued in some way.
You might have previously seen goodwill on a balance sheet, or something of that nature. But what you’re saying now is that there’s a material and quantifiable value of data assets which, if you pair with the previous sort of, “Well, it’s growing exponentially,” then maybe the deal is all about collecting the data if in fact we can execute against it. A growing percentage of the value comes from the data less than from the human or physical elements.
David: Absolutely. It stuns that we do multimillion dollar projects in migrations and consolidations, and if you ask that question, what we just discussed, half the VPs and the CXOs that are funding those projects can’t answer that question. That, to me, is stunning.
It also is a shift in paradigm thinking about, well, what do we need to do to justify this? If you think about the reams of data that you were alluding to that haven’t even happened yet and that we can’t even understand the impact of, how can this data culture not exist? That is the foundation for a data driven company, right? It should be.
Ledge: We’re probably five years, it’s not even that long, away from where the AIs go ripping through everything and all of us just sit back like Wally and we drink a smoothie in a chair and float around.
There’s a lot of enabling forces that I think …. In the conversations of having a lot of AI leaders, the big thing everybody focuses on is going to be the, ‘well, this is about human augmentation, not replacement’.
David: Yes, agreed.
Ledge: I don’t know. What do you think around those things? I think it’s difficult to picture a business where you don’t have to do the things that many of us do now.
David: Agreed. I guess the augmentation part, I would really phrase that and re-message that. That I think it’s about the extended efforts that we can do.
In the example I used before about autonomous vehicles, the mechanic isn’t going away. She may be going to do something else. The Feds/SLEDs funds that are fixed amounts of SLED zero sum game, they’re not going away and disappearing, they’re going to be reallocated to something else.
In terms of the actual human impact, I would suggest and argue that the overall impact isn’t positive, it’s just a redirection of the effort level and concentration. Maybe an example I would think of that like comes to mind because it’s been in the papers all the time is, retailers lose about 8% of their revenue because, I walk in, I want Cheerios, there’s no Cheerios, then I have to buy cornflakes, I say, “Screw it, I’m out of the door.” Or I want a Red Delicious apple, they only have Granny Smith, so 8% of the revenue is lost by that.
What they’re try to do, of course, is put cameras everywhere and figure out when I grab a box of Cheerios and it’s no longer on the shelf. Then because other retailers are actually doing fulfillment out of the retail stories, this pressure is a calculable, real revenue loss that they’re trying to find a solution to, and AI is an obvious example of that.
If I’m a data scientist and I’m taking all their Cube 64 cameras, putting all the data in, 50 cameras in a store and scrubbing it up this pre-calc stuff. I put it into these data models. I’m scrubbing it down to the RGBs of 264 bits. I can calculate, in this example, just this exact example where no one is losing their job, it’s just being re-vectored to, go back to the warehouse just in time, put the box back on the shelf based on new information that they’re calculating.
It’s not a net loss – because I’m kind of an old thermodynamics guy, entropy never goes away. It’s not a net loss, it’s just re-vectoring the current activities to be augmented by the dataset. Not augmenting my activity, per se. My activity may go away but another one will arise.
Ledge: I love that. The thermo and your chemical engineering background coming out there. It’s the preservation of matter and energy indefinitely, just in a different configuration.
David: Yeah. Another example that kills me because I’m a football fan. The LA Rams moved back to LA from St. Louis. They’re building this huge stadium down there, and it’ll be this integrated work-life, drink, sleep, complex with the football stadium, theaters. It’ll have retail. It’ll have restaurants, bars.
If you think about an application of huge datasets in AI, when I go in there and I’m a frequent user of Chili’s Restaurant and knows that, every other establishment will know I’m a frequent user of Chili’s and what my preferences are. So, that actual person asking what my preferences, that goes away, but the actual person fulfilling that preference – because I’m buying a Kate Spade bag for my wife but I don’t like blue, I want red. Can I see what red looks like? And they flash it on a VR/AR screen and they fulfill it the next day, that’s a new activity. That’s a net created job that didn’t exist before.
Yes, some things will go away but the net creativity I think will increase, to your point, exponentially depending on the data.
Ledge: It’s a good vision. I like reading about it. Let me ask you this. Tactical things here. You get to, obviously, think about big ideas. Take yourself back to career development up to this point and tactical success factors in your career, in your technology career.
How could you advise people who are out there, in our case writing code and deploying servers and all those things? What are the behavioral traits that allow you to be a big thinker and to apply that in a meaningful way to the business?
David: Now that’s a great question. It’s a question I ask a different a way to people I hire. Is that, do you want your hands dirty? You can’t run a business, know a business from sales to technical, if you’re not willing to get your hands dirty and do the business and know the business.
I find a lot of people like to say they know it but don’t really understand it, and that’s hard work. It’s getting your feet on the ground. It’s actually coding. It’s doing all the things that you thought you maybe had left, but to really understand the pitfalls and the successes and where you’re strong. There’s no way to do that without understanding it deeply. For me, I find I have to do it.
The example I’ll use is kind of a success and failure, so it was a dual-edged sword.
I ran a team in Africa. Twenty-two people. We sold OEM for Microsoft. I thought I was this really smart guy coming from the US and I could do it because I did it in the U.S. I got my lunch handed to me. The first six months I didn’t know what I was doing. I didn’t how the channels worked. I wasn’t communicating effectively to my team because English was a second language to everybody.
I had to really step back and refresh, who am I? What am I doing? Do I really know what I’m doing here? The answer was no, I didn’t.
I spent three months to four months, I visited everyone, I went on sales calls with those guys. I understood how they really used the tools. What they didn’t like about them. The culture was very different – the Northern Sahara, Sub-Saharan Africa, Middle East.
It was a humbling, humbling experience and rewarding at the same time because we blew out our numbers. But we couldn’t do that until I failed, and it was me failing, not them. I had to recognize that I was that throat that needed to be choked, so to speak. Once I did that, everything was fine. But that self-realization, I think, is also very, very important.
Ledge: The humility then of learning.
David: Humility. Always.
Ledge: “I don’t know.” The three most powerful words. I always like to say, follow that up with, “… but let’s go find out.”
David: Yeah. I would add right before that, “I’m sorry. I don’t know. Let’s go figure it out.”
Ledge: Absolutely. That’s a great insight. I don’t think we can do better than that.
I’m going to say, thank you, David, for joining us today.
David: Yeah. It was my pleasure and anytime, Ledge. Thank you.