Machine Intelligence and the Future of AI with Oren Boiman of Magisto
A 20-year veteran of software engineering, Oren Boiman’s Bay Area-based Magisto has reached 100 million users over the last decade. Oren has been a visionary in his field, understanding the importance of AI well ahead of the curve. Magisto is a pioneering video editing app, which employs AI to assist with the editing process.
This fascinating chat with Ledge covers the future direction of AI and user interfaces, and the importance of the younger generation ‘needing to know’ everything!
If you want to harness AI in order to better understand customers then this podcast is a great place to start.
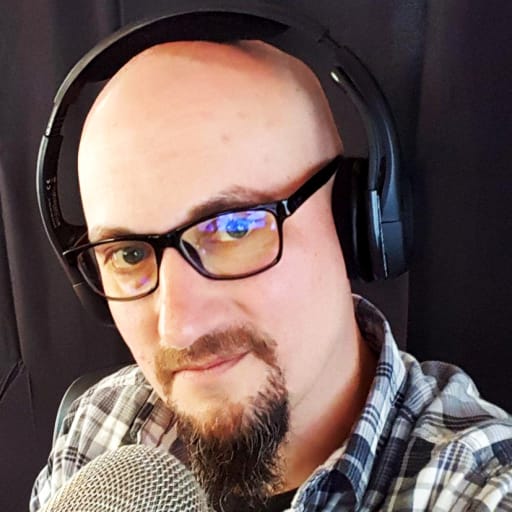
Transcript
Ledge: Oren, it’s great to have you. Thank you for joining us today.
Oren: Thank you for having me.
Ledge: Could you just a couple of minute background story of yourself and your work, and tell us about your firm?
Oren: Sure thing. I’m Oren. I’m the CEO and Co-founder of the company called Magisto, which is an AI-based app and platform for video creation. It’s actually AI to help to create videos.
I’m coming from a very technological background. I did my major and master and a PhD in computer science and mathematics.
Specifically, I was drawn to computer vision and AI early on. That’s where my passion for AI came from. I also had a product management background which got me very interested on what AI can do to products. I’m talking here about a couple of years ago before AI was a topic that everybody talks pretty much everywhere. Where we ended up is with Magisto having an app and platform that has served over 100 million users, and about 100,000 paying businesses and subscribers.
It’s going to be interesting that, we got to a point where it’s becoming obvious right now that AI is so embedded in products that what we thought years ago as vision, me and my co-founder Alex – also a PhD in Computer Vision and Mathematics – is now everywhere. Our kids are expecting to talk to the computer. It’s so obvious. Which is interesting to think about. I would not imagine about the future, what the future’s going to look like if this is where we are right now.
Ledge: Absolutely. My kids, you can’t even say, “I don’t know,” anymore. That used to be the great answer. But now they say, “Well, just ask Google.” You can’t not know.
Having been out in front of that, what has this experience been like when the rest of the world caught up, and pop technology is using the things that were in your head before and you actually have a real business around it? Help me sort that out because there is so much misinformation about AI now, and you’re actually doing it.
Oren: It’s a pretty difficult thing to do. There’s a lot of hype about AI, but at the same time there’s this huge kind of ever-forward motion with AI innovation. It’s like you read about that in the papers almost every day or every week, which is pretty amazing. But at the same time, there is a very big gap between AI in the academy or AI in research papers, to AI in a mass scale productized version.
It’s something that early on we learned how challenging it is. Some of the things that we became better and better is in understanding that the product management of AI is something that is, I think, only now starting to be appreciated.
You can see the gap. If you look at how much innovation there is with regard to AI and how much money is being poured, compared to actual products that me and you could touch – and we just talked about the Alexa/Siri versions of them that now we’ve got as interfaces, and there are product and specific niches like integration as Magisto.
But, given all the hype and everything that we’re talking about, it’s actually not a lot. There’s a gap there.
The ability to productize AI I think is creating a substantial obstacle. We also see that in self-driving cars. In the implication of it, there’s an inherent tradeoff between letting the AI do its thing to controlling that. It’s a very, very difficult tradeoff. The tradeoff is actually the most important point where the product needs to make decisions.
Let’s take an example, for instance, for an assistant type of things. If you assume that the AI is always correct, and that was case, that would be very easy. The AI will understand what is the query or what you’re trying to do, and you take that, you parse that, and then you give an answer. But then, how do you backtrack when the AI doesn’t get it? It’s actually very, very difficult.
It’s all about the entire product. The product is not about what happens when you get everything right, it’s about what you get when you don’t get it right.
So, the product about how to deal with the fact that AI might not understand the intention, or the fact that the user wants to adapt it, or not all information was passed, is something we sometimes ignore. It can be a lot of the emphasis we put into products.
For example, when we built Magisto for the first time, it was intended for consumers and it was fully automatic. You gave Magisto photos and videos, you pressed a few buttons, and then you got a video back. This was kind of the deep understanding of everything that goes into storing characters and everything. But you couldn’t change it.
So, let’s say you said, “Okay, that’s nice but it’s not what I intended.” How do you communicate that to the AI. Now you need a different level of interfaces and innovation just to be able to converse. Not by talking but by some form of interface – it can be an API, it can be a UI – to explain and direct the AI where you want to go. If you can do that, the benefits of doing that are immense because you’re essentially turning people into Superman.
With Magisto, we can take the fitness instructor, yoga instructor, that are very good in being yoga instructors but they don’t understand anything about video. They can create video within five or ten minutes as professional video makers – and do that in 10X less time than it would take to a professional video creator because they’re using manual tools.
It’s a huge advantage, but the product has to be able to solve for the need to communicate with the AI and to direct the AI to where you want. That’s a crucial thing. Again, I think it happens where the AI makes mistakes or sometimes for instance when you talk about assistants. When you talk about assistants, there’s a lot of commonsense when we speak that is not there. There’s really not in the query. It’s not the AI’s fault. The AI needs to know everything about the world and what you’ve done and where you’re coming from and where you live to know what’s happening right now on TV or whatever. The context is missing.
In order to direct that, this became some of the innovation in recent years. That there became an ability to build on context. So that’s a form of user interface which lets you guide the AI to where you want, and understanding that those gaps in information need to be filled with products.
So that’s essentially where we took it, and we have tens of patterns which we learned the hard way by being the first and trying to break various roles. Some of them were technological, some were just we didn’t know how to make people express their intentions in a way that AI can understand.
That’s something that, in many ways, is happening everywhere where you have AI and everyone needs to solve their AI product interface questions in a different way.
Think about self-driving cars. Assuming that you can control the car and this is not a fully autonomous car that does whatever it wants, how do you control it? What happens if it doesn’t really understand what you wanted? That’s in real time where things are moving and everything.
These are all things that need to be solved for every product that makes use of AI. I think that’s where the sophistication and technology is driving us.
Ledge: There’s all kinds of stories about Microsoft unleashes their AI on Twitter and it learns in 48 hours how to be an awful racist. It’s all about that input. I imagine that along the lines of your user journey on your productization, that you also learned how to turn your users into the trainers of the future experience, right?
So you’ve got two different tracks there because each user in that interaction, if done well, trains it to be better for the next time.
What are some of the stories along the lines there? I don’t think you started chunking out racist videos, so tell me some of the stories that were mindboggling on the way to getting where you are.
Oren: First of all, you talk about the learning aspect of the artificial intelligence. Obviously, that’s a very, very important one. That’s what really moved AI from five years ago to today. It’s really about learning, and a lot of it is deep learning, the ability to use massive amounts of data, which is interesting.
In our case, we started from a B2C offering. So as a leading video creation app in the App Store and then the Play Store and everywhere. This is where we started from, where we had a better understanding of the customer because we are consumers and dads and we also communicate on social media. We understood what it means to be able to create a video for personal expression, and only later we moved to add businesses and professional video marketing aspects of the product.
To begin with, what happened because of that path is that Magisto got hundreds of millions of videos produced on the platform, and that created invaluable data. Which, probably if we chose a path which just goes through a B2B offering, we would never get those volumes. Never. It’s kind of interesting that we got there without really intending, because I think that the aspect of the amount of data and how crucial it was to get a mass amount of data, was just being more and more established in the AI research community as AI improved.
It was not the same thing seven years ago. The AI, deep learning revolution happened in significantly less time.
It happened to be that we made the right choice and we got the data. The data significantly improved our ability to get to extremely high accuracies, and also to get indelible data that relates to things that don’t exist in databases. So you can have databases and repositories about face detection and object detections and all those kind of generic activities of computer vision and AI, but how would you find a database of hundreds of millions of videos, what is regarded as the best video storytelling? There’s no grand truth for that. It’s really about people’s tastes.
By generating that data, it gave us a huge boost in order to improve the product. As it’s grown, we use more and more of those abilities to drive innovation.
The thing there is that, actually it’s not that similar. When you’re looking at that from a machine learning perspective, you have a very nice enclosed problem of; you have a problem that you need to solve, you just put data, you do it by doing number crunching and you get some sort of a network blackbox that just does the work and nobody really understands how.
When you’re working in a real situation, again there’s a product there and there are users, it’s very, very different. As you are improving your capabilities the users do what users do, which is they come up with requirements. Those requirements are sometimes not there. They’re not even optional.
Again, when we started Magisto, it was a fully automatic process. Now, suddenly when users say, “We want control,” there’s what we talked before about the product question about how you even integrate that control, how you input that control. Then, once you have that, you train something and now you need to be able to poke it in multiple ways – something which is black box-ey in many ways – and try to move it to the places that the users want it.
So, there’s another level of learning that happens for every startup. This is the product/market fit where you get feedback and you create features. But when you have an AI engine in the middle, that AI needs to adapt by itself. It’s not the same function that just learns more and more and more data. It’s becoming a completely different beast over time. It complicates things tremendously, but the benefit is you’re giving even more and more inputs.
With Magisto for professionals and businesses, businesses can get the initial video – what we call a draft – and they can get inside and then start to play with it. You’re playing in almost like a storyboard problem where you can shuffle things and say, “I want to highlight that,” and have captions.
That creates a lot of options for interface. Every one of those interfaces you have a user that says, “I got what I wanted,” or, “I want to change it.” Which is more and more inputs. So the app becomes better and then users comes with new requirements.
It’s not just the improvement of AI with data. This is a function and a black box thing that will expect that just runs and improves itself, for something like a module for face detection. When you have a user with an interface to the AI, it starts many, many levels of cycles of improvement. We always find those things inspiring because you always learn about new features and new requirements from the user. Then what happens is that, as you try to plug those understandings to the product and the AI, you’re creating new problems in AI. A lot of the problems and a lot of innovation that we did in Magisto are actually problems that are unknown as important. Nobody’s working on that in the academy.
I’m sure that’s true for every domain. For self-driving cars. It’s, as you solve those things and as you try to improve the way for people to control and harness the power of AI, then you’re creating sub-problems that are crucial as you go, and nobody knows about them. So the question is, do you have data to solve them? How do you solve for them? Is it good enough? When you solve it, what kind of other residual problems that are remaining? What are the new requirements with users?
This is fascinating. These are the unmapped and unchartered territories of where AI is taking us. That combination between product user and AI, it’s and the product/market fit when you take AI and put it there, it’s a three-way conversion between technologies, new technologies, product requirements and customers that at the end of the day are always right. It is fascinating.
I think that most of our innovation is from there. Not just trying to be innovative in doing a better object recognition or tracking whatever. Obviously, we have tons of data and we do that on state-of-the-art level, but the real interesting things happen when you’re getting to the details of their domain. Where you really are solving, for the first time, crucial problems that nobody even know exist.
I’m sure they are, again, on speech and assistants and self-driving cars. Everywhere has its own thing that the people that really work deeply on that understand these are the interesting problems. Not just understanding the phonetics and understanding the word. It’s much more than that.
Ledge: Yeah. It makes me think that the inevitable path to the general AI is really everyone doing all of these successively less narrow applications and inputs. It’s the collection of all of these narrows together. It’s almost like the limit that we may never approach is the combination of all things.
Oren: It’s so far that it’s not easy to see even how we get there. I would say it’s much further. For people that are afraid about that, they can be afraid about real things like AI taking jobs – which is true for every technology. Any automation technology can take jobs. That’s real and that needs to be attended to, but the general AI, no. That’s not even close.
Many of the leaders of AI, the big companies, they’re participating in those conversations but inside they’re saying, “What are we even talking about? It’s not even there. We’re not even doing the basic stuff. What are you talking about?”
Ledge: Well, it captures the zeitgeist. Everybody wants to know when Skynet is coming.
So, let me switch gears a little bit because you’re obviously, I can tell from just talking to you, you’re a passionate explorer and researcher. That’s the path that you came from and yet you have to wear the CEO hat.
I just wonder, how do you make space for both worlds? Being a CEO is a full-time job and you don’t get to be a researcher as much and scratch that itch. In every measure of engineering and science, we see that. Where people are making tradeoffs in their life to lead instead of to be able to crunch code or algorithms or research.
How do you make that balance?
Oren: Well, first of all, as you say that balance is hard. Also, I think that the thing which is hard about that balance is that it changes over time, a lot. What we’ve done when we’ve been a team of 10, and what we’ve done with a team of 20 and then 50, it changes all the time and you have to adapt to it.
The first thing is that you really have to have a team of ninjas that can do the work by themselves. It’s my co-founder and our leadership team and the people that are recruited. We enjoy having a really, really talented team that has stayed with us for a long time. You learn over the years to develop processes that are almost telepathic. You don’t need to even formalize them, it just happens by itself.
In many ways, I was able to step out more and more and more from the process because many of the things happen by themselves. It’s becoming unconscious for the CEO because they happen and then you don’t need to intervene.
Where it’s still important, and this is where my role in this happens a lot for founder CEOs, they fill the role of the product vision. Vision is a big word, it’s more like direction. Let’s go this way. Where are we heading in terms of direction? The thing which is important for AI-focused products is that it’s very, very easy, very easy to point in directions that are simply impossible.
Actually, if you think about that, there’s a narrow crack somewhere between technological feasibility, product need, enough customers, business model that makes sense. When you’re taking all those constraints together, there’s kind of a tiny crack. The ability to read that crack and not point everybody to all the rest of them which are just walls, this is critical. It’s true for every startup, but even more so with AI because there is much more in AI we can’t do than the thing that we can do. Or even think that potentially you can do them but they’re going to be so expensive, they’re not going to make sense. There’s not going to be a business case for doing them.
My role is really about the ability today to see where, as CEOs should do, the market is going and what things in market trends and trying to foretell the future – or least foretell the present. Even if you can predict the present, given the pace of change, it’s something. Then directing all the experts in our company to find that crack. It’s amazing how small that crack is. It’s so easy to take directions there that are not feasible. Which is true for every business.
Think about the majority of success stories we hear about startups. Usually we hear about success stories about startups that were sold so fast for so much, but it’s actually before they developed a product which people wanted, but they didn’t have a business case or a business model.
Getting to a functional business model is drastically more difficult than just getting a product out there which people like. Then, adding on top of that competition and the way the market changes and technology changes on top of that, which changes a lot and fast, I think this is where I’m trying to go with the company. I think I’m doing it in more and more directional way and I cannot, even if I really want to, intervene in the day-to-day questions of research which are fascinating. They’re just fascinating.
That’s what I like about the topic, because it combines not just the AI technology but also creative research. When it comes to video creation and marketing, it’s about the ability of people to express themselves. These are also things, if you think about video, now video has reshaped social media and now we’re taking about the videofication of the web. Video reshaping the internet.
Those things are also innovations. How people communicate and their effectiveness. All those things are working together and the advance of AI. It’s really fascinating and fun and I have to stop myself in many places not to dig too deep, just because it’s so much fun and, as you say, I have other duties to do.
Ledge: Well, I love that. I love the passion. I’m sure we could do this all day long because I’m fascinated by all of this.
Oren, thank you. I think what you guys have done and created is really exciting. I hope everybody goes to check it out. We’ll make sure that they have links and all of that.
Great, great story, and thanks for joining us today.