Interview with John Dubois, co-founder of Oculus360
John Dubois is the co-founder of Oculus360, joins Ledge on this episode of the pod to discuss the AI space, and how Oculus 360 is using AI to change the game on generating consumer insights for brands.
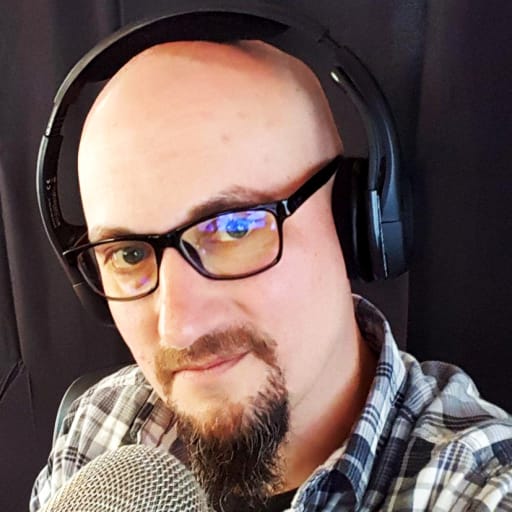
Transcript
Ledge: John, great to have you. Thanks for joining.
John: Hey, thanks for having me, Ledge. Awesome to get to do this together.
Ledge: It’s nice to rip on some nerdy stuff together. Could you, if you don’t mind, give a two, three-minute background story of you and your work, just so the audience can get to know you a little bit?
John: Sure. I am an engineer by training and CEO by day. I work with Oculus360, which is an AI platform that is used to decipher what’s intended in terms of purchase demand from comments and pictures and other things that consumers may upload.
I’ve been doing it since 2012 and been an entrepreneur since ’97. A little bit on me.
Ledge: Right on. Good journey there. We were talking off mike, it’s just like, AI for marketing and consumers. Wow. There’s only like 7,500 companies that talk about that now in their copy.
But you talked about the plight of the trailblazer, originally. When you have to be the person that makes a new category, or the company that takes responsibility for that, and all the bumps and bruises.
Tell that story a little bit because I know a lot of people resonate with that.
John: Sure. In 2012, if you think of just even the way consumers engaged was different. So your data source is different in this case.
When we were first going to market talking about, yeah, from consumer comments you can extract when people are using products, and what they think about it, and what are the best features for those occasions, we were getting a lot of looks like, “That sounds fantastic, but I don’t know where to put you guys. Is this social listening?” Well, no, we’re not really social listening. “Is it really just hardcore market research? Are you just sending out surveys?” No, we’re not doing any surveys. We’re observational. We’re just listening. We’re just interpreting what people are saying online, and we’re teaching models, training models and machines to do the interpretation.
It was really tough.
Ledge: Was it disbelief? Like, no, that won’t work, or… I don’t know. If I’m in your seat I’m going, “I don’t care what budget you put it under, just buy it.”
John: Exactly. Exactly. The thought that, yes, this sounds too good to be true. If you’re not having humans read this and train machines what to do, then it sounds magical. Like, how can you actually be doing this without supervising the machines?
So we were taking some pretty hardcore machine learning, a little bit of neural network and some vectors and applying it to this problem. At the time, people were still like, “But where’s the thumbs up, thumbs down from your favorite social listening platform?” We’re like, no. You can get emotions. You can get so much better than that. You don’t have to settle for that.
But it was a tricky time. I think having big companies like Google, Microsoft and IBM enter into that space has helped because small companies like ours don’t have to do the education any more. Now people just understand there’s a need and value for it.
Ledge: That’s such an interesting point because I think there’s probably an equal number of people that are terrified – rightly so – that such and such big company just entered my space. I’m so hosed.
But, I don’t know. Maybe it’s about really knowing your niche and understanding the difference between the weight of that educational category-defining factor. Did you have to adjust to the way that they were training about it and change your way of discussion? Were the words different?
John: That’s a good question. I think it wasn’t so much that as, we have a patent pending around occasion extraction. What this is is similar to Google’s micro-moments, or it’s similar to these things.
The difference is, theirs is based on search intent and ours are based on comments. You can point out how the artifacts that someone is getting, or deliverables someone is getting out of a platform are similar, but that the approach or the data that is being studied is different. Then you present why that’s different, or why that’s important.
So search intent is great for getting intent, but not so great for getting what did they think of it after the purchase. So there’s a difference.
I think of it, these guys – to use a Star Wars analogy. You’ve got Star Destroyers which are kind of blazing the trail, but when there’s a problem you’ve got to send out TIE fighters. It’s the little guys that are out fixing the problems, settling the smaller battles, but it’s the big guys that are helping everybody move along. I think that’s kind of what’s happening in this industry.
Ledge: Absolutely. It’s interesting hearing you talk about the unattended AI. I talk to a lot of AI companies and experts, and there is not a large segment of the AI and ML utilization that can exist without human involvement and intention that does make the model smarter.
I know particular cases where you’re just like, well, we need such a high degree of positive mapping that we do want to have a human intervene so maybe you don’t have to run into that. You’re not trying to save somebody’s life, for example. Something like that.
Talk about that. What have you done there on unattended? Just the story around that.
John: In this space of machine learning or training, whether that’s related to NLP or word vectoring or other things, you kind of have the whole supervision, which is human supervised training, and then semi-supervised. Which is where you where you may do a little bit and then let the machines figure out the rest. Then there’s unsupervised where you just throw out a data set and it figures out patterns out of that. Neural networks are an example of that.
Our approach to scale and to enable our predictions and extractions in any consumer facing industry forced us, basically, to figure out, how do we get a machine to train itself by just looking at the data?
So the first problem that you have to solve is, you need relevant and clean topical data, because if you’re building models on something you need to be less fuzzy and less dirty than you might if you’ve already trained something or you’ve curated something manually using industry expertise.
So, our goal is to take consumer commentary that we know is related to a topic – and we’ve got a set of algorithms that help us determine that even – and then use that to model out industry taxonomies. So it’s coming straight from the consumer.
The thinking is that in marketing, in R&D, in sales, if you put the consumer in the middle of your strategy then you’ve got something to really anchor onto. When do they use your products? Why don’t they use your products? Maybe there’s something missing. So from an R&D standpoint that’s valuable. When do they use it is valuable from a marketing standpoint. How do they use it is great for sales.
So, if you can get machines to study those comments and even the imagery, then you have a pretty good win.
One of the keys to the way we go to market is, our platform sort of daisy chains a bunch of things together. After NLP and some of our machine learning algorithms run, the outputs of those become the inputs of the next step in the process.
It’s completely hands-off, but you can look at it as we’re using the output of some machines to train for the input of other machines using other algorithms. It’s a pretty cool technique that you haven’t seen a lot about. You see a lot of companies in the space that are really strong at NLP, are really strong with neural networks or image recognition, but you rarely see one that can just study the comments and use the text in those comments, relate it to the image, to train on how to decipher the image. It’s just a cool space to be.
Ledge: So, where are all these comments? Is it social media? What are the sources for what you’re putting into it?
John: We have an intermediate data format so we can use social media. We only study publicly available comments, so Twitter is more powerful in that case than Facebook because Facebook is a lot more private now.
Ledge: It depends on who you ask, right?
John: Then you’ve got blogs. We do blogs, we do forums, we do product reviews. It’s a really rich data set. If you map out what each of those data sets are used for, then you can start to model what’s the consumer journey.
On Twitter they become aware of something via marketing or via influencer. In a review they might be talking about why they’re considering two options. Like, I really like this about these running shoes but I prefer this about these other running shoes. So you can kind of understand when they become aware and how they consider options.
In commerce you get purchase, a little bit of purchase, and behavioral.
Then in forums you get this rich texture. If I’m talking running shoes and you want to know what’s the latest trend in fashionable running shoes, you could say, well, fashion maybe you follow some Instagram influencers and you see what running shoes they’re… But if you’re actually a runner and you’re running marathons and you have shin splints after Mile 13, probably not going to get that in reviews. You’re probably not going to get that reading Twitter. But you probably will get it in a runners forum.
Ledge: Letsrun.com.
John: Exactly. So that’s the idea. Is, if you can pick up these signals and tie them together across all these, even if you’re not tracing the same person, just a similar type of people, you can start to understand that decision journey better.
Ledge: Right. So, talk about an actual case study. Particularly, my brain goes to, tell me things that you figured out that were totally non-intuitive and blew minds when you came to a conclusion.
John: It’s marketing, right, so we didn’t find… We weren’t able to visualize the first black hole. But in marketing, for one large US auto manufacturer, we were able to tell them the Achilles heel for their competitor in the same division was the placement of cup holders. You’re like, how’s that mind-blowing? Well, the fact is it was targeted at soccer moms. With this bit of information they ran spring sales event and all sales event campaigns, and had pretty overwhelmingly good results. Triggering some of the…
Ledge: Just by talking about the cup holder.
John: I know. It’s like something you’re like, really? But it’s things like that or…
Ledge: All the industrial designers are like, dude, we just spent two years on the body design and they don’t care.
John: Exactly. We already have 12 cup holders, why do I have to move it? That kind of stuff.
Ledge: I’m a father of five. It’s the first thing I check.
John: That’s awesome. I’ve got one but he’s young so no cup holders for him yet.
We had a brand that was stagnant – this is in the vitamin space, working through one of our agency partners. It was stagnant and they were trying to figure out what happened between product launch and today?
We were able to diagnose that it came down to a change in marketing message which was brought on by some over-cautious perhaps legal folks that said, you should stop saying that you’ve put fruit and vegetables in your capsules to make the capsule body of the vitamin, because we do but it’s such a small percentage.
So they went back to that and they saw positive results. They got credit for something they were already doing but had to stop talking about, not for any real legal reasons but for some over-cautious reasons. In the end, they were able to sell more product.
Like I said, it’s not the black hole but it’s some cool stuff that you can tease out of this.
Ledge: Absolutely. Just technology wise I have to ask. This sounds like this workflow that you’re talking about, chaining together all the inputs, outputs. Certainly you’re in some kind of cloud environment at this point. I imagine that, with some kind of monstrous migration from on-prem or bare metal since 2012.
I don’t know. Any good stories there? I’d love to know where you ended up. Obviously, a lot of people want to get into this kind of space now.
John: We started in AWS and are still in AWS. Our footprint has grown. We’ve done some things to modularize things using Docker containers and Ansible and other things that are maybe not native AWS – they’re more open source or more best-of-use, best-of-breed stuff. That’s enabled us to pull in other artifacts as needed.
Yeah, it’s really pretty core. We’ve used some of the native AWS stuff, obviously S3 and that sort of stuff. But even at one point I believe we were using RDS, which is their version of relational database – PostGres I suppose – and their version of Elasticsearch. But in some cases the way that those things get configured out of the box don’t really fit your case. So you end up rebuilding it on their , on AC2 instances.
So, yeah, we’ve been there for a while. We’ve got an automated environment using Ansible, some Jenkins. We do use a little bit of CloudFormation for certain areas. Lambda functions.
Ledge: I was going to say, you clearly have a use case for serverless, and that would have been something that came available in the middle of your lifespan. You probably reduced your bill quite a bit by doing that.
John: For sure.
Ledge: I mean, hundreds to millions of transactions that you can create and destroy. I imagine that you had a lot of efficiencies to be gained there.
John: Oh, for sure. We had a full EMR cluster doing things that we were able to retrofit in Lambda with really very little effort. All of them we were using things like Pig and other stuff, so you have to obviously rework that. But the efficiencies and the costs, monthly costs for the same type of work has gone down. Yeah. It’s been pretty fantastic. I recommend that stuff when it can be done.
Ledge: Absolutely. What’s your engineering org look like? I mean, you must have to iterate the product just on an absolutely constant basis. How do you deal with that?
John: We’ve got different leads over each area. So we’ve got a UI built-in node and an API for that. We’ve got someone that owns that. We’ve got a deep data science history and team there. We’ve got data collection and API consumption – so another person. Then infrastructure. It’s those four groups for the core platform.
Then we’ve got folks doing customer delivery and the other stuff like that.
Ledge: Your data consumption, you talked about having intermediary data formats. So you’re going to build into your taxonomy there. I imagine that you must be in a constant treadmill of data sources that you need to normalize. So, I guess ETL going actively insane there?
John: Homegrown ETL, but we’re looking at Glue and some other tools in that area. So Glue and another Amazon tool that I’m not as familiar with that allows you to query from flat file and then [00:18:35] transforms. Unfortunately, I’m not as well versed in that area.
But yeah, definitely ETL is important for us to get everything into a format that we can… As I said, if your daisy chaining all of these things together, you need to know what all the inputs and outputs are. Even at the beginning of the pipeline, we have to make sure the inputs are formatted correctly.
Ledge: Video must be monstrous. Are you consuming video too?
John: We’re not doing video. We do stills currently, and mostly the stills we’re analyzing are product listing images. So, pretty straight forward – what’s the sleeve length, is this a V neck or not? That kind of stuff.
Ledge: Right. Think about product reviews. It’s like everything is an un-boxing video now. There’s so much stuff there.
John: Absolutely. Yeah. That’s just the next big thing, right.
Ledge: Talk to me about, just from an organizational standpoint since you said you wore a lot of the executive hats. Compare and contrast what those roles were like, and being a technical leader and then having to be in the CEO seat.
I just think there’s very distinct differences between those, and how you navigated that.
John: This is my fourth organization, and in the previous three I was in the CTO role. That’s definitely a natural role for me. I think when we started Oculus360 in 2012, I took on operations – which is something I hadn’t done before so I was intrigued with it. I think a year-and-a-half into the journey I then moved to taking on the CTO role, which again is kind of my heritage.
I think if you just compare and contrast the two, operations, contract review, legal – all the things that us as developers are not that fond of doing but it needs to get done, it has to get done. If you delay getting an invoice out then you’re going to delay getting paid, right? Brief answer. We all understand that. You scale that in an organization, it’s kind of a big deal.
Ledge: Let me tell you, the contract review process is such a peach. Yes.
John: Exactly. A friend of mine named Matt Clark , he used to say, it’s the best work that no one wants to do. That’s kind of that operations role.
You move into technology and I think that’s just a blast because you’ve got one eye on what you’re doing and one eye looking, trying to look around the corner and see what’s next. Ultimately, the goal in my mind as the CTO is to keep the business and the solution at a point where you can still charge a premium for that service.
Whether you’re a freelancer and you’re doing your own training and you’re kind of playing that role, or you’re in an organization and you’re worried about pressures from offshoring and other things, it’s best to have an eye on what’s the market demanding, and what are they willing to pay top dollar for? How can I take advantage of that?
That, to me, is a lot of fun because you get to go to… You have conferences. You get to meet a lot of cool people and discuss how new tech is going to affect current or today’s situation.
The CEO role is new to me, and this is also a venture funded company so it’s my first time also having to deal with venture folks. That’s been eye-opening and quite a learning experience. But in this case, it’s now not just technology decisions and solution decisions, it’s decisions around hiring and decisions around where we should invest. Should we do this data agreement? That kind of stuff.
It’s more holistic. I think for me as an entrepreneur for so long, one of the hard things to do is learn to go a little bit hands-off and delegate and trust the team. Now when you’re faced with a role that you have your hands in a lot of different things, you absolutely have to delegate and be comfortable with the team that you’re delegating to.
That’s kind of my…
Ledge: That’s absolutely right. Trust is one of those things that you kind of either do it or you don’t. It’s so easy to micromanage, because you’ve been there, you built the thing. You did all the stuff. The original code probably came from you.
John: It did, yeah. All the stuff not used anymore came from you.
Ledge: Right. All the stuff that is now legacy that your devs want to throw out is yours. Your bad comments are being trashed.
John: Just a quick story there. I have one of our data scientists, just a fantastic guy – actually the whole team. I threw out a problem. I said, this is great. When we were first tackling image recognition – which for us it wasn’t recognize whether there’s a soccer ball, it was recognize whether the soccer ball is plastic or leather and what the primary and secondary colors are. So it’s feature based. It wasn’t just, this is an object – which you can do with a lot of APIs.
So, when we were testing this out we had to first start with manually curated labeled data because you’ve got to make sure your algorithms are going to work.
I was saying, well, maybe let’s look at TensorFlow and let’s look at these other tools – ConvNet and other tools and toolsets. I went off, was doing my thing, checked back in. The team was like, yeah, okay. well we were able to do what you said but when we did it this other way we got 98% accuracy in this.
It was, okay, I didn’t mean do what I said. This is great. I’m so proud.
That’s the sort of moment where you’re just like, you’ve got to learn to trust and let go. Especially this generation that’s growing up digital native with taking coding in school, which I mean we had TRS-80s when I was in high school. That dates me a little bit. Coding was a little different. We were doing Basic.
These guys, they’re learning stuff that is just so phenomenal that when you ask them to apply what they know to a problem, you just got to… If they need direction, fine. But otherwise just kind of let them at it and you get great stuff.
Ledge: That’s fun. I can hear the passion in your voice to that, so that’s good. You must be on the right road. Very cool. Very cool.
Well, John, this has been a great conversation. Thanks for joining and sharing the insights.
John: Absolutely. Pleasure to do it, Ledge. Hope to talk again soon.